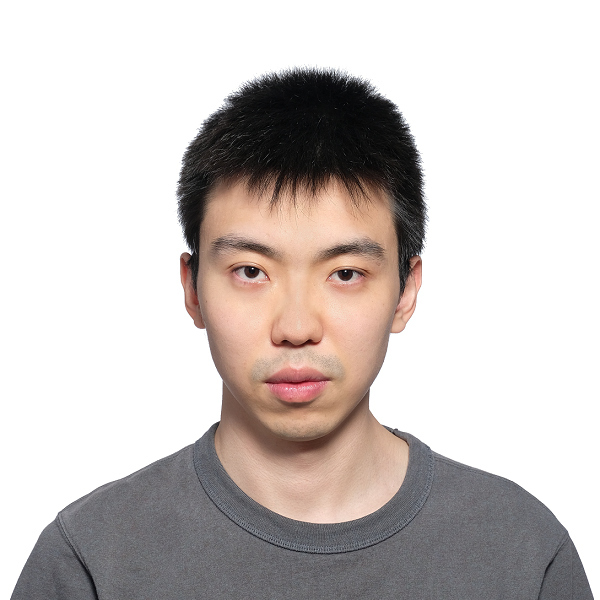
Bio
I am a third-year Ph.D. student at Computer Science & Artificial Intelligence Laboratory (CSAIL) at MIT, supervised by Prof. Wojciech Matusik. Before coming to MIT, I received my M.Phil. from the Chinese University of Hong Kong, supervised by Prof. Dahua Lin. I got my Bachelor's degree from Tsinghua University.
I am generally interested in computational design problems at the intersection of geometry processing and machine learning. My current focus is on combining the expressivity of procedural modeling and machine learning for the generation and inverse design of graphs, with a wide spectrum of applications to molecules, smart materials, and, more notably, 3D shapes. By means of developing high-performance optimization algorithms and frameworks, I am excited to work with everything that is visually impressive and practically efficient.
Publications
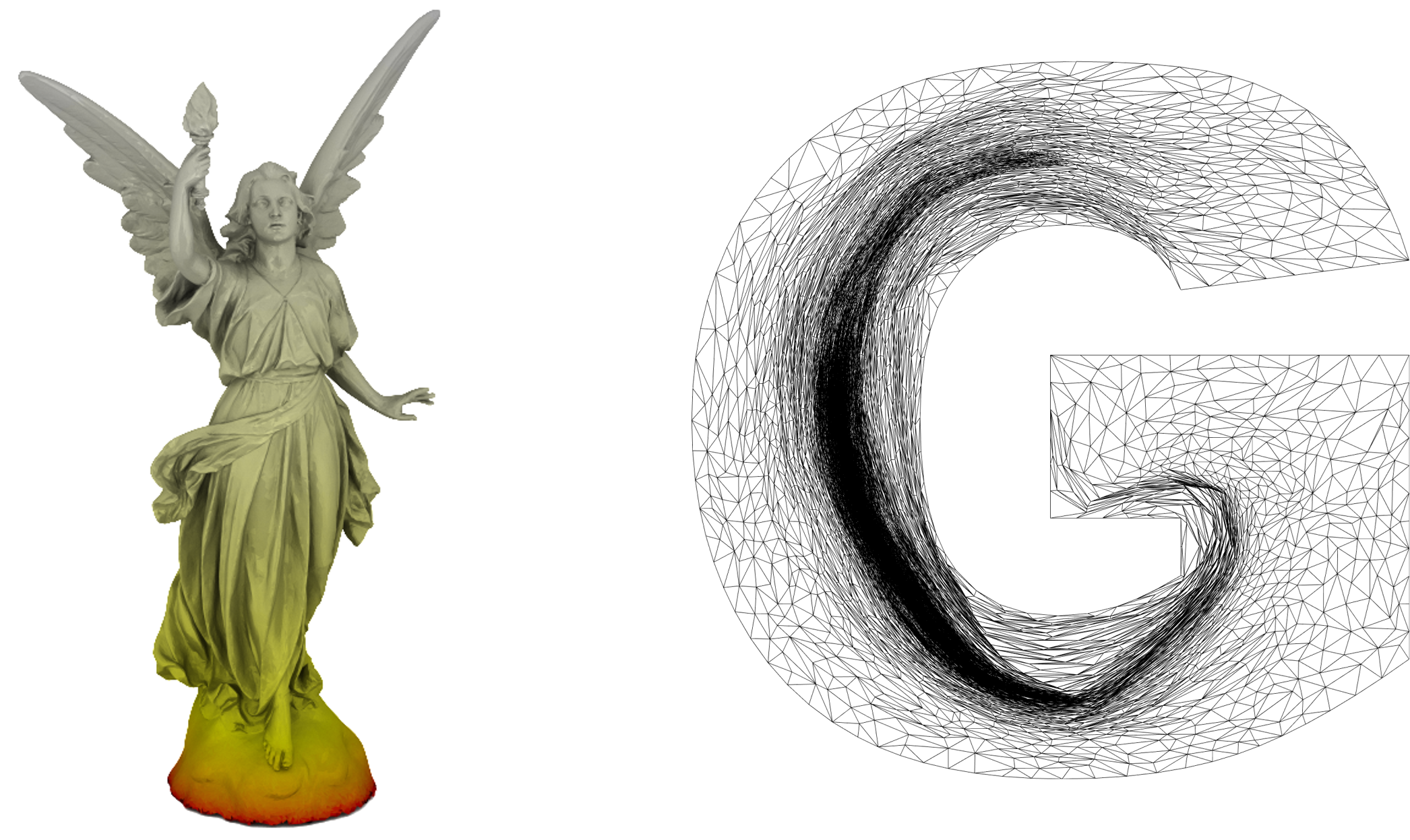
Variational Quasi-harmonic Maps for Computing Diffeomorphisms
Yu Wang,
Minghao Guo,
Justin Solomon
ACM Transactions on Graphics 2023 (SIGGRAPH 2023)
[PDF]
Abstract
A novel method for computing smooth, inversion-free maps based on variational quasi-harmonic maps and a special Cauchy boundary condition. By transforming the mapping problem into an optimal control problem defined by an elliptic PDE and minimization within a family of functionals, an efficient numerical procedure is proposed, yielding significant improvements in speed and quality over current methods and allowing for optimization of a generic energy in the framework of quasi-harmonic maps.
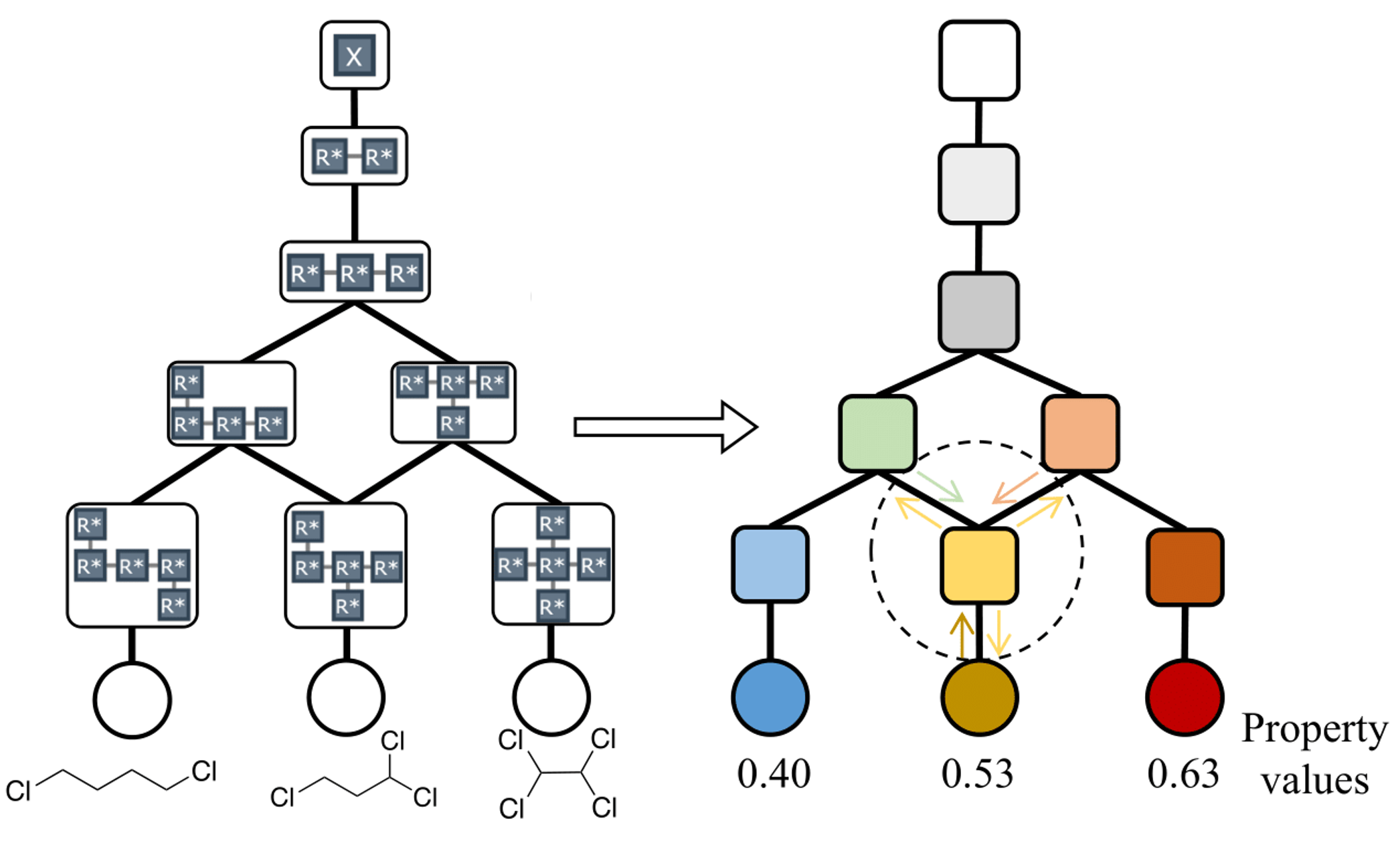
Hierarchical Grammar-Induced Geometry for Data-Efficient Molecular Property Prediction
Minghao Guo,
Veronika Thost,
Samuel Song,
Adithya Balachandran,
Payel Das,
Jie Chen,
Wojciech Matusik
International Conference on Machine Learning (ICML) 2023
[PDF] [Code]
Abstract
A data-efficient property predictor for molecular properties utilizing a learnable hierarchical molecular grammar and graph neural diffusion over the grammar-induced geometry. Despite limited labeled data, the proposed method significantly outperforms various baselines, including supervised and pre-trained graph neural networks, in both small and large datasets.
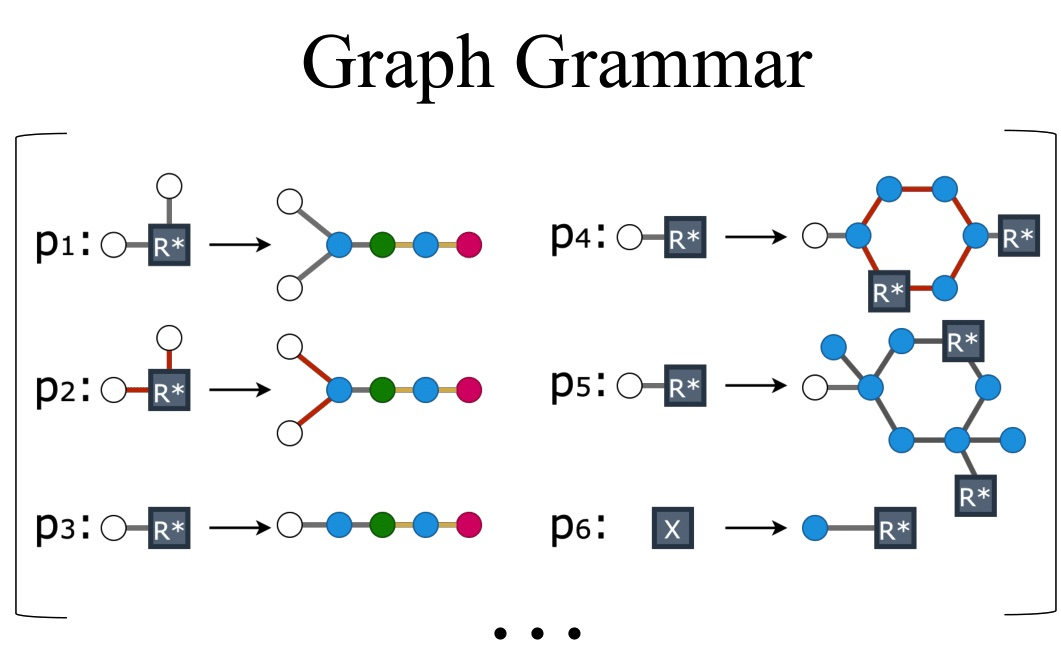
Data-Efficient Graph Grammar Learning for Molecular Generation
Minghao Guo,
Veronika Thost,
Beichen Li,
Payel Das,
Jie Chen,
Wojciech Matusik
International Conference on Learning Representations (ICLR) 2022
Oral Presentation [acceptance rate: 1.6%]
[PDF] [Code]
Abstract
A data-efficient generative model that can be learned from datasets with orders of magnitude smaller sizes than common benchmarks. At the heart of this method is a learnable graph grammar that generates molecules from a sequence of production rules. Our learned graph grammar yields state-of-the-art results on generating high-quality molecules for three monomer datasets that contain only ∼20 samples each.
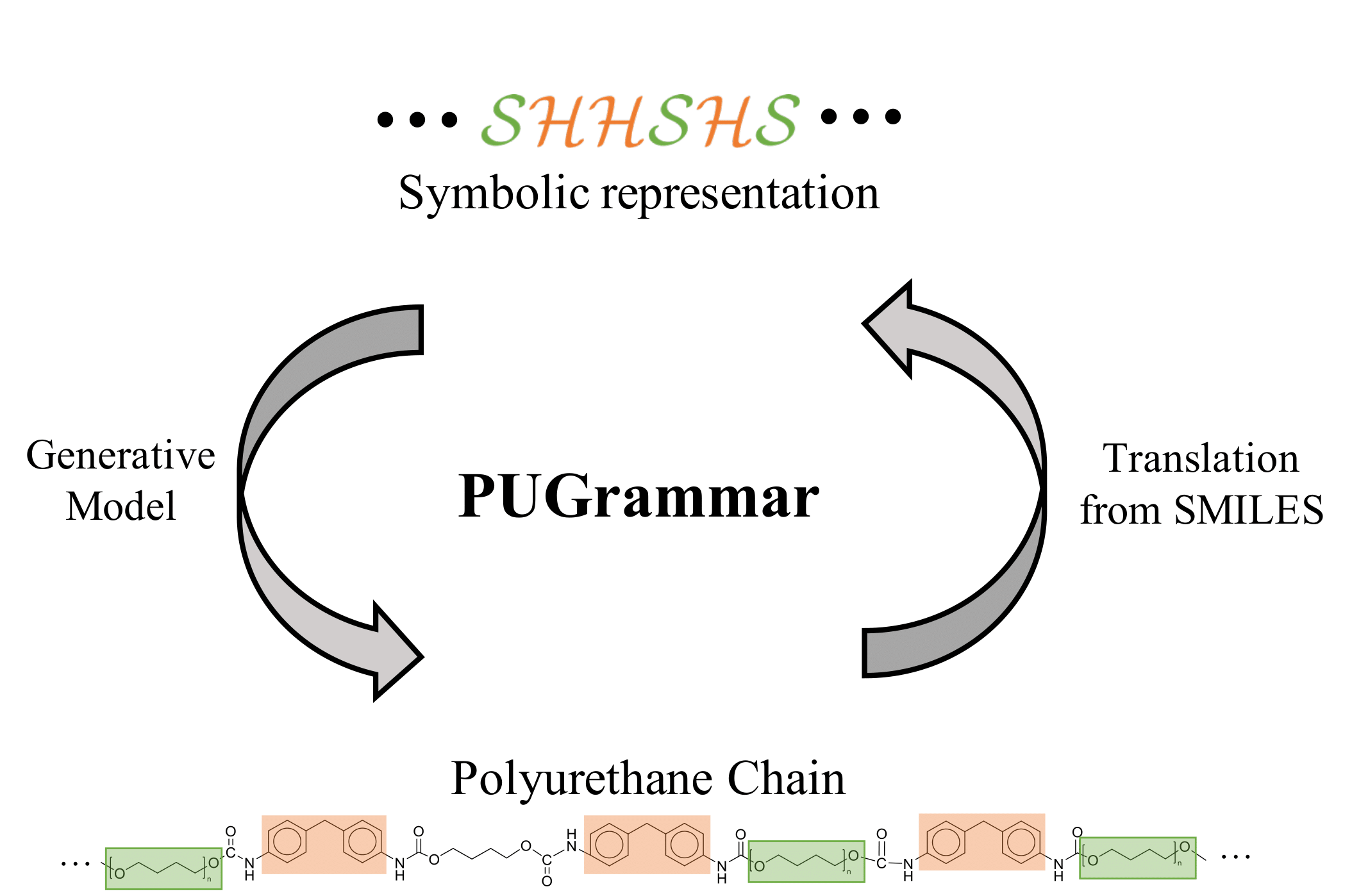
PolyGrammar: Grammar for Digital Polymer Representation and Generation
Minghao Guo,
Liane Makatura,
Wan Shou,
Timothy Erps,
Michael Foshey,
Wojciech Matusik
Advanced Science
[PDF]
Abstract
A formal grammar specifically designed for the polymers, providing a concise, explicit, and robust representation for any polymer within a given class. This project serves as a foundational blueprint for constructing general chemical design models, with closely integrated representation and generative capabilities.
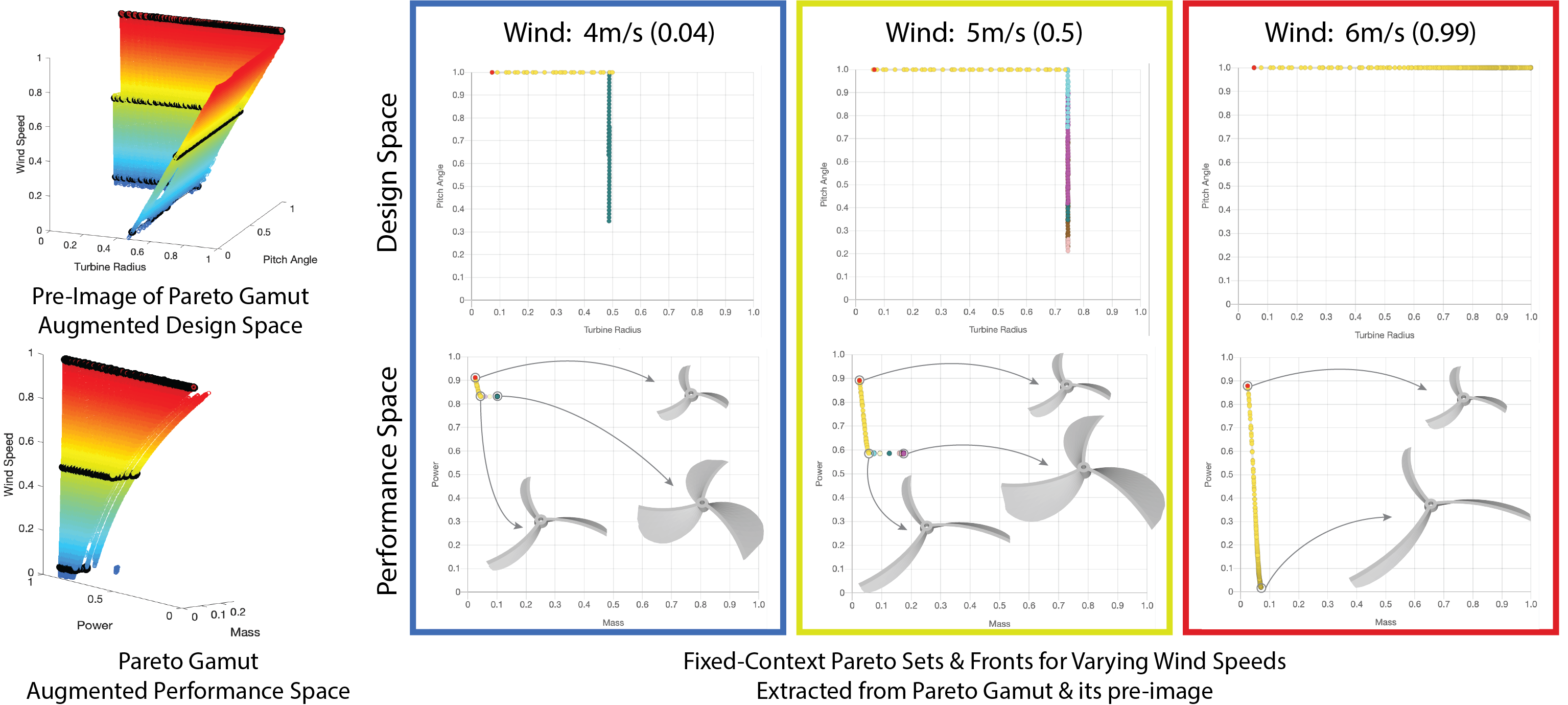
Pareto Gamuts: Exploring Optimal Designs Across Varying Contexts
Liane Makatura,
Minghao Guo,
Adriana Schulz,
Justin Solomon,
Wojciech Matusik
ACM Transactions on Graphics 2021 (SIGGRAPH 2021)
[PDF] [Project Page] [Video]
Abstract
A framework for variable-context multi-objective optimization capturing Pareto fronts over a range of contexts (Pareto gamut). A global-local optimization algorithm is developed to discover the Pareto gamut. Its practical utility is demonstrated for several engineering design problems.
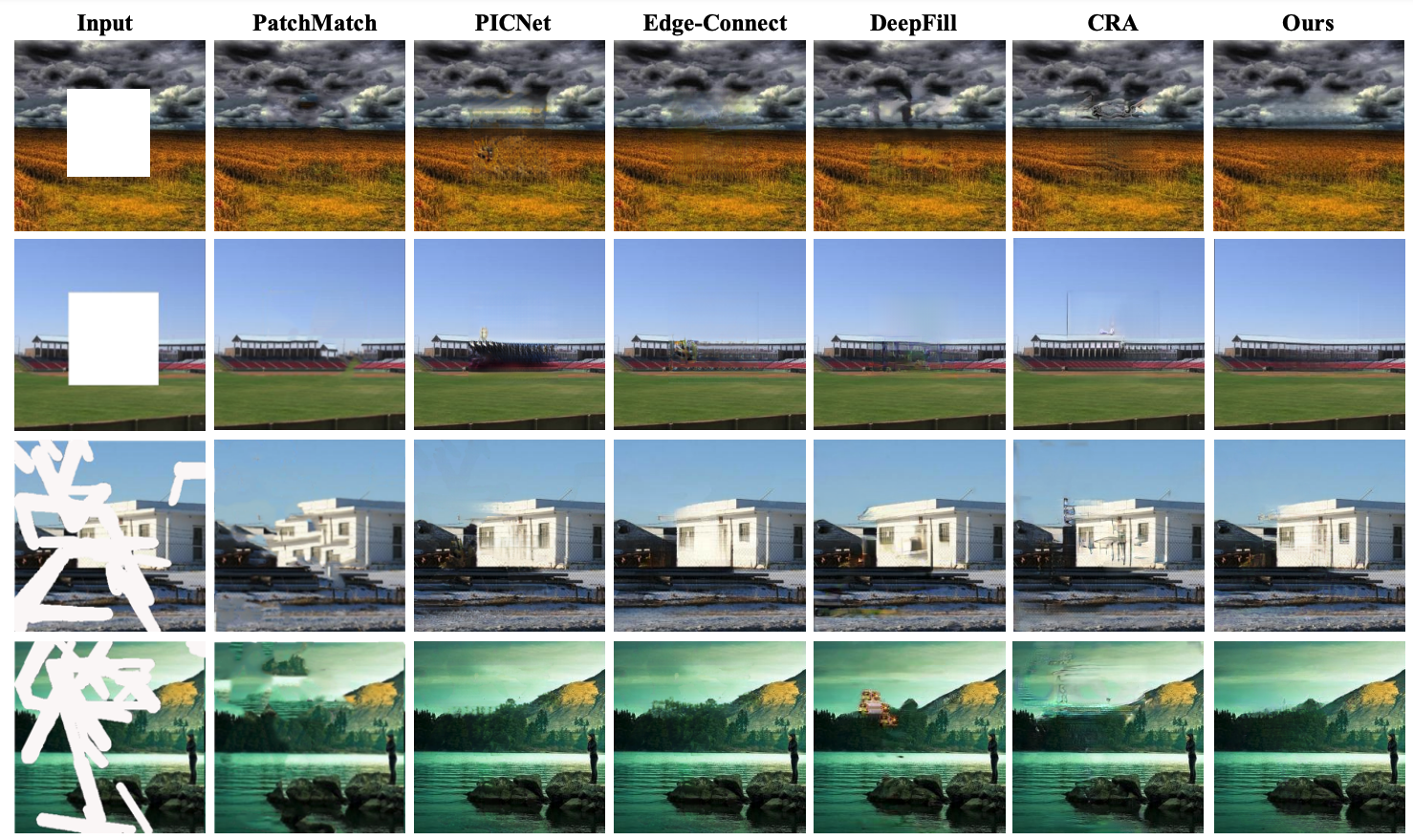
Texture Memory-Augmented Deep Patch-Based Image Inpainting
Rui Xu,
Minghao Guo,
Jiaqi Wang,
Xiaoxiao Li,
Bolei Zhou,
Chen Change Loy
Transactions on Image Processing (TIP).
[PDF]
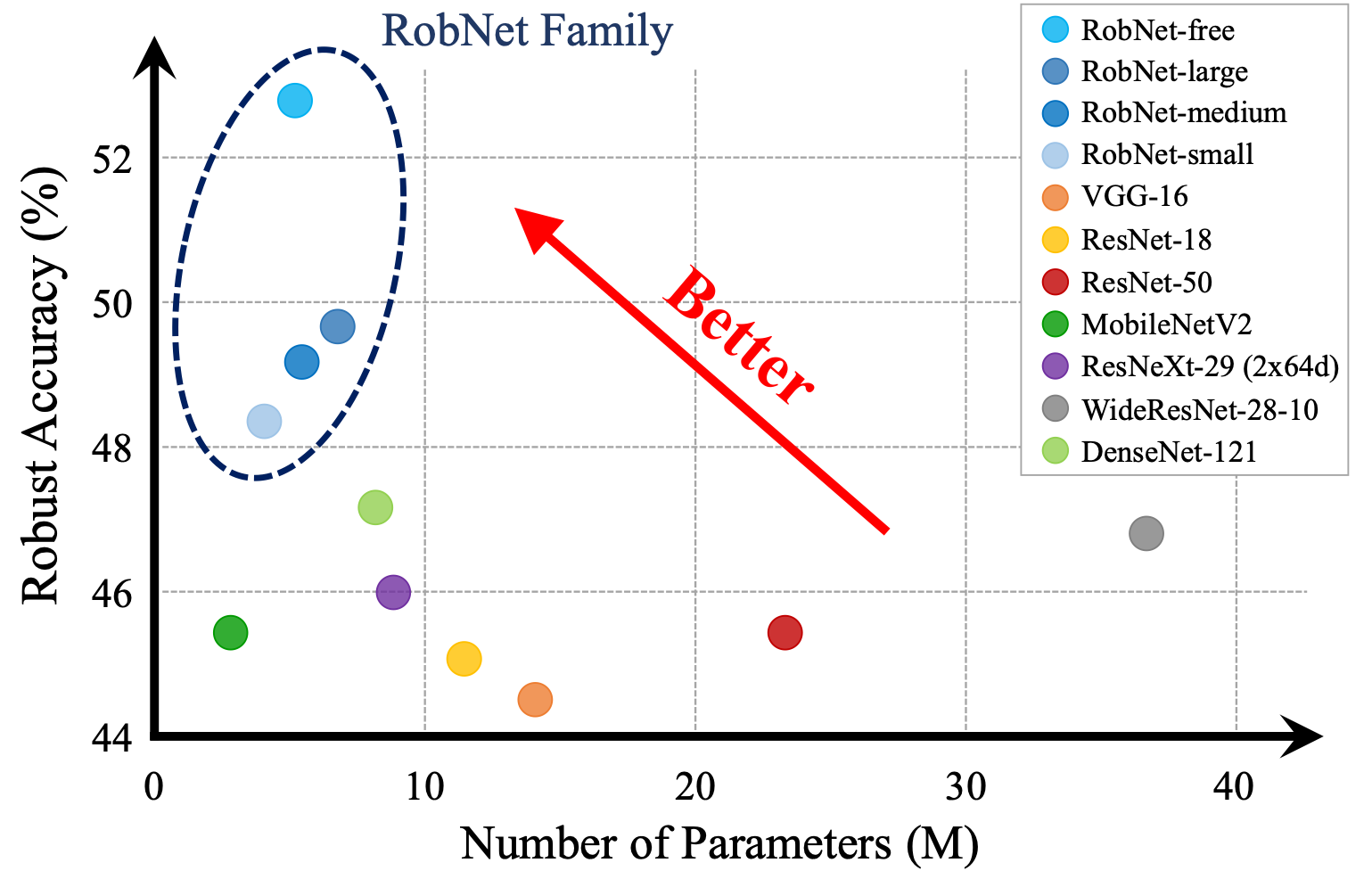
When NAS Meets Robustness: In Search of Robust Architectures against Adversarial Attacks
Minghao Guo*,
Yuzhe Yang*,
Rui Xu,
Ziwei Liu,
Dahua Lin
Conference on Computer Vision and Pattern Recognition (CVPR) 2020
[PDF] [Project Page] [Code] [Video]
Abstract
Take the first step to understand adversarial robustness of neural networks from an architectural perspective. Based on our “robust architecture Odyssey”, we design a family of robust architectures RobNets that exhibit superior robustness performance to other widely used architectures.
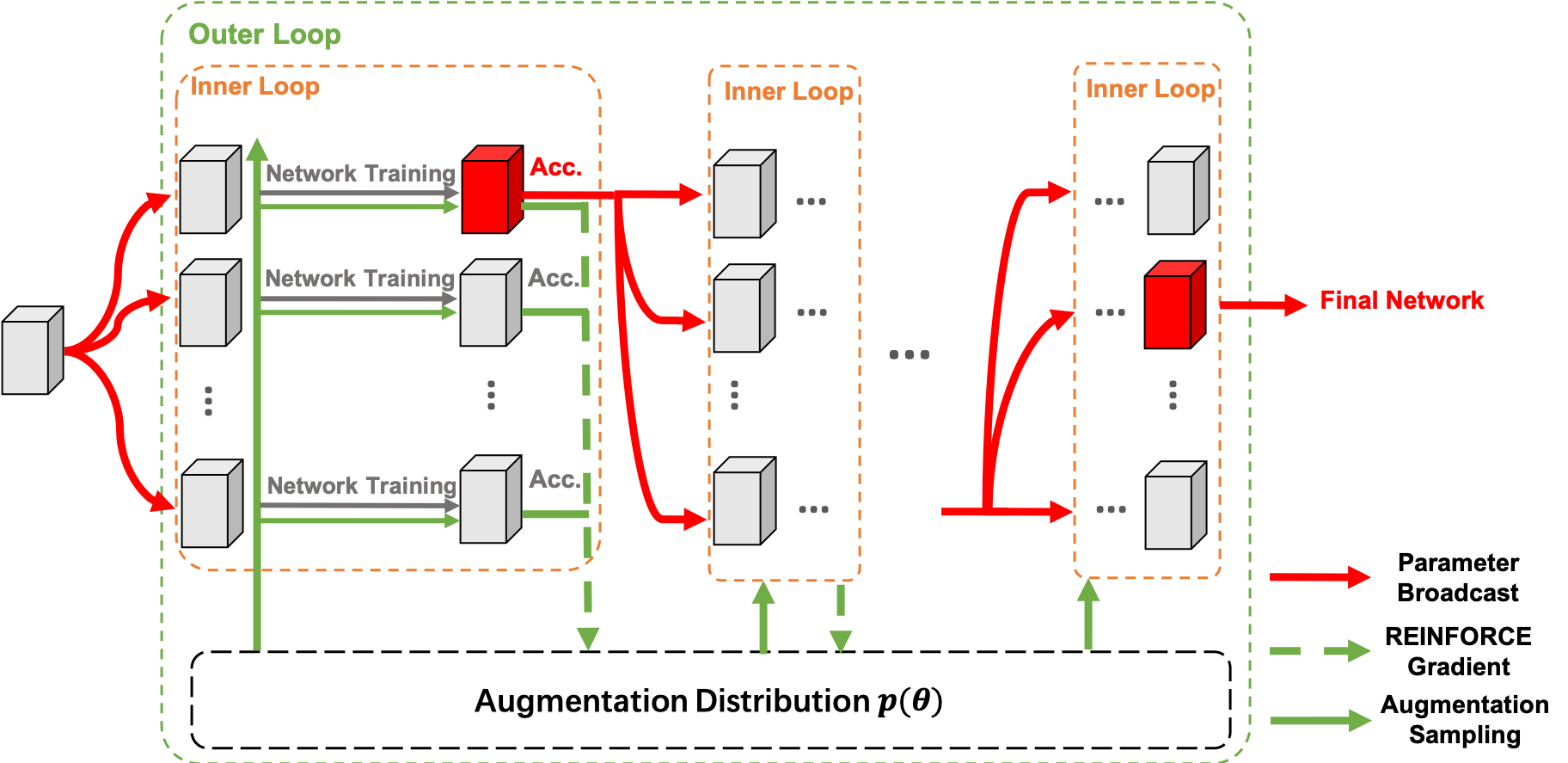
Online Hyper-parameter Learning for Auto-Augmentation Strategy
Chen Lin*,
Minghao Guo*,
Chuming Li,
Xin Yuan,
Wei Wu,
Junjie Yan,
Dahua Lin,
Wanli Ouyang
International Conference on Computer Vision (ICCV) 2019
[PDF]
Abstract
An economical solution that learns the data augmentation policy along with network training. The augmentation policy is formulated as a parameterized probability distribution, thus allowing its parameters to be optimized jointly with network parameters. Compared with SOTA methods, this framework achieves 60× faster on CIFAR-10 and 24× faster on ImageNet, while maintaining competitive accuracies.
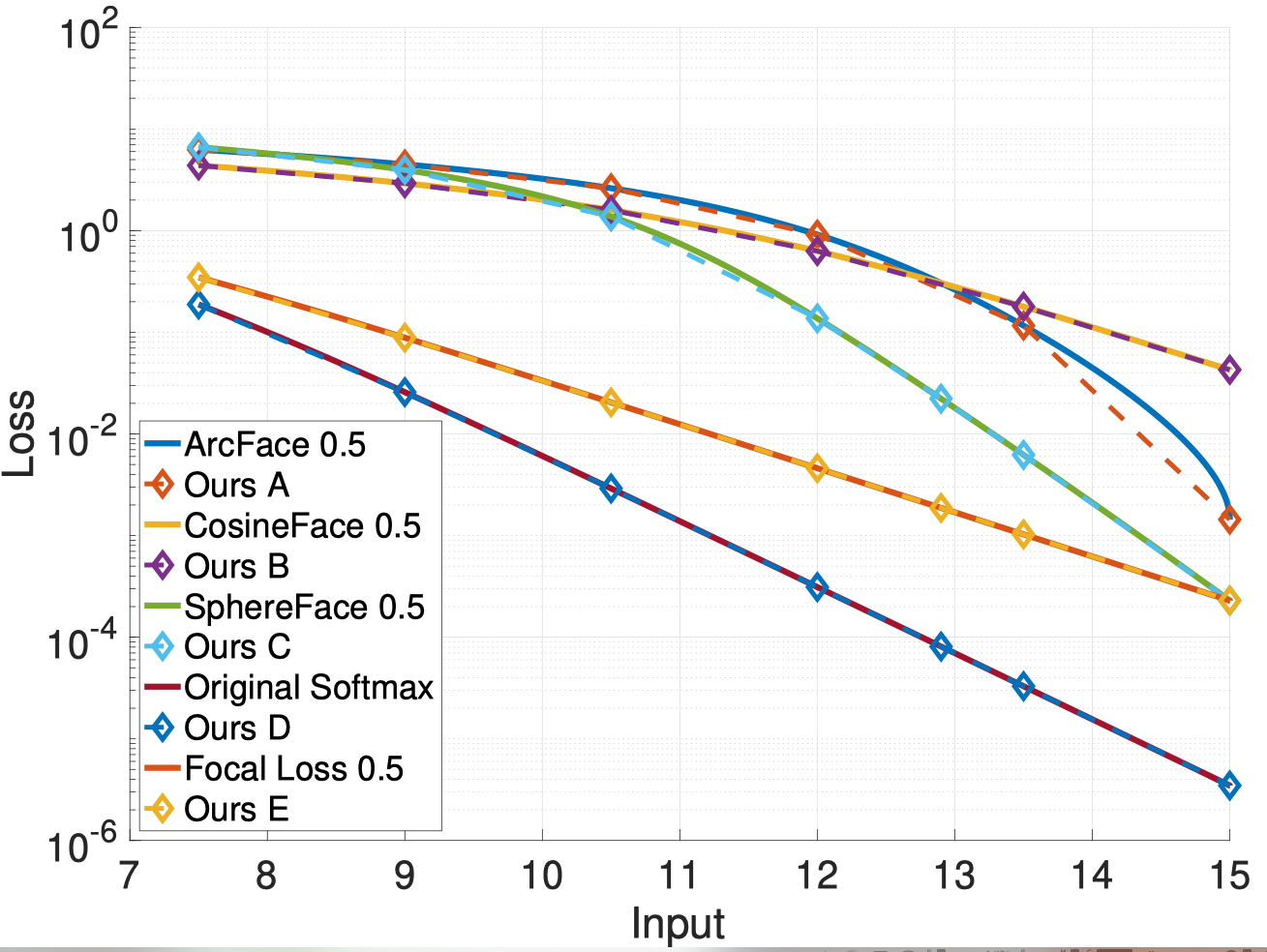
AM-LFS: AutoML for Loss Function Search
Chuming Li*,
Xin Yuan*,
Chen Lin*,
Minghao Guo,
Wei Wu,
Junjie Yan,
Wanli Ouyang
International Conference on Computer Vision (ICCV) 2019
[PDF]
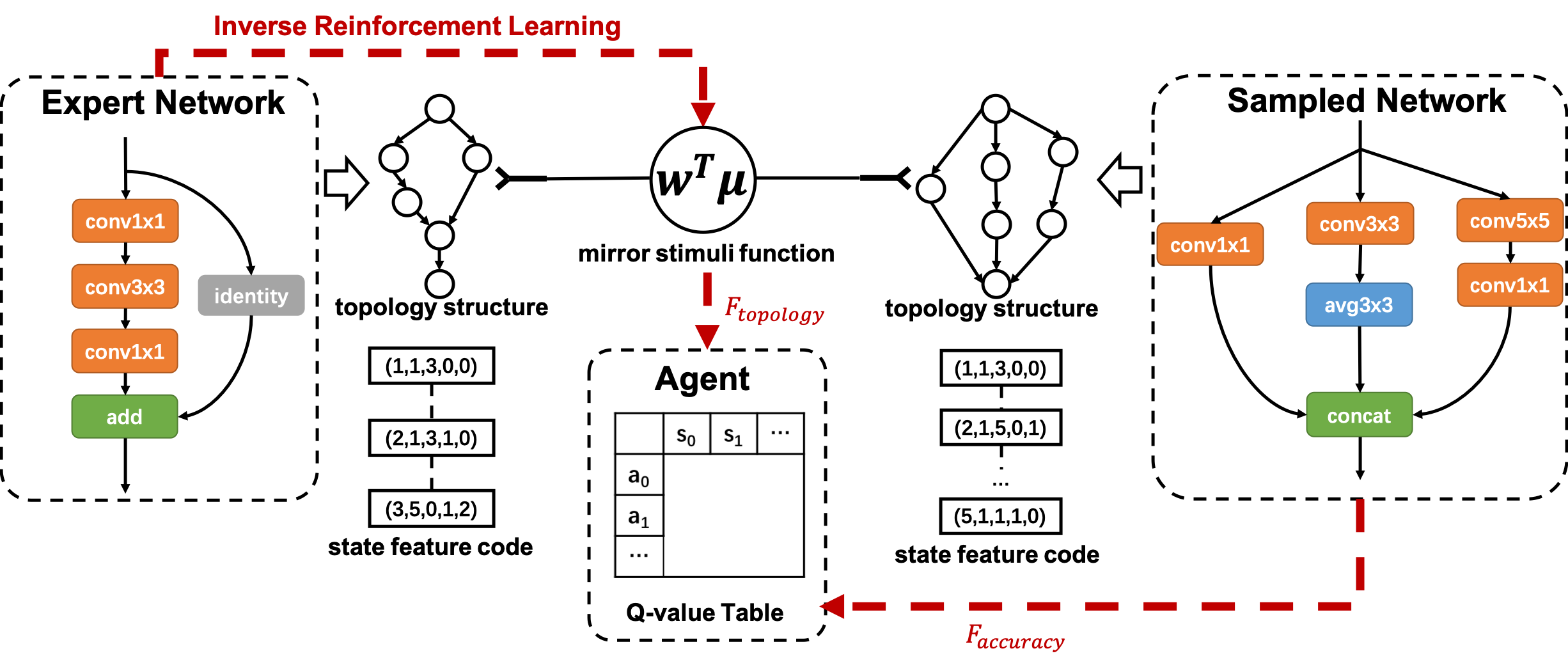
IRLAS: Inverse Reinforcement Learning for Architecture Search
Minghao Guo,
Zhao Zhong,
Wei Wu,
Dahua Lin,
Junjie Yan
Conference on Computer Vision and Pattern Recognition (CVPR) 2019
[PDF] [Code]
Abstract
An inverse reinforcement learning framework for the training of an agent to learn to search network structures that are topologically inspired by human-designed network. A mirror stimuli function motivated by biological cognition theory is proposed to extract the abstract topological knowledge and facilitates the discovery of architectures that stride a satisfactory balance between accuracy and efficiency.
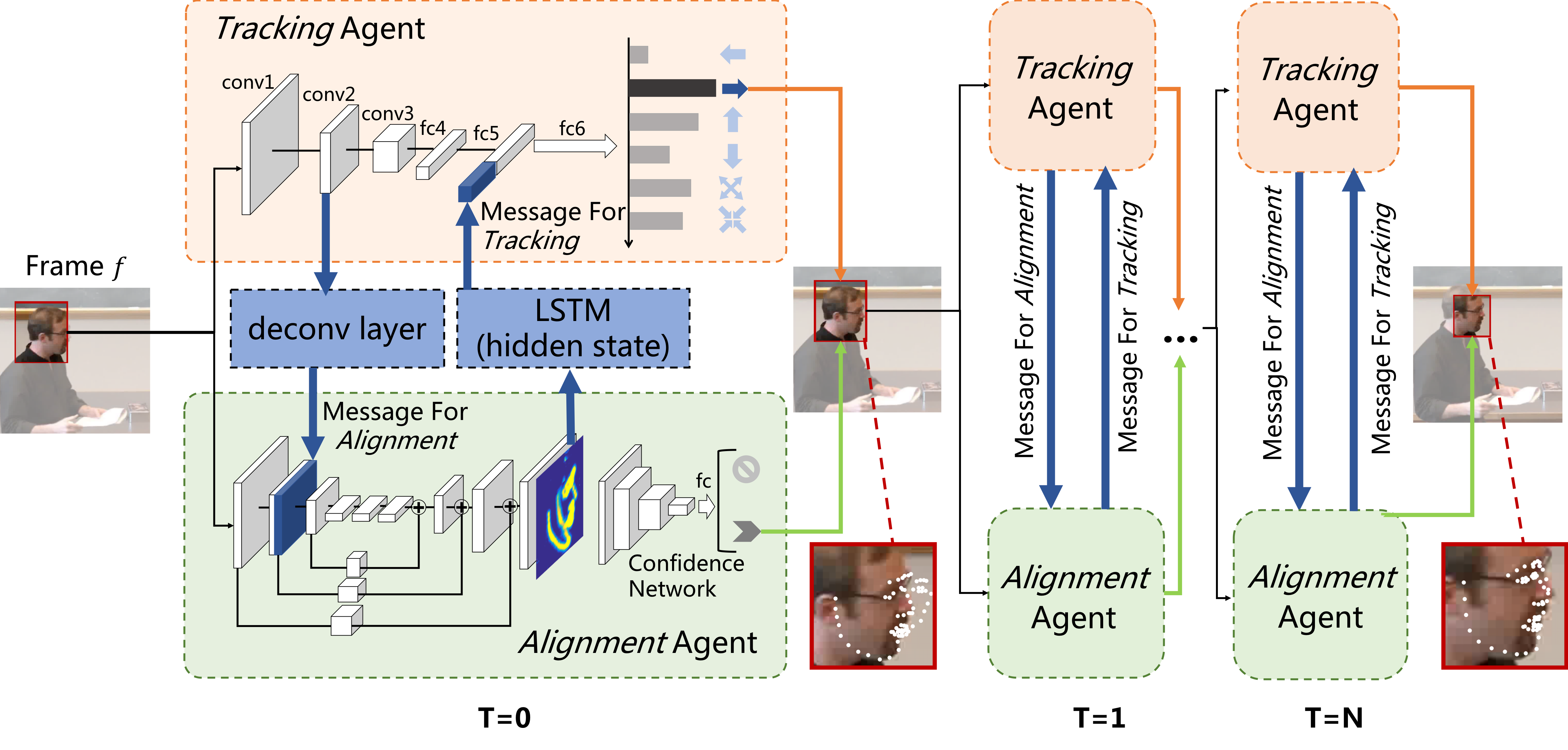
Dual-Agent Deep Reinforcement Learning for Deformable Face Tracking
Minghao Guo,
Jiwen Lu,
Jie Zhou
European Conference on Computer Vision (ECCV) 2018
Oral Presentation [acceptance rate: 2.4%]
[PDF] [Video]
Abstract
A deformable face tracking system that can automatically balance the efficiency and effectiveness according to the image quality and tracking complexity of each frame. Dual-agent deep reinforcement learning is used to make adaptive decisions to position updates of bounding boxes and landmarks. The interaction of these two subtasks is explicitly exploited following a Bayesian model.
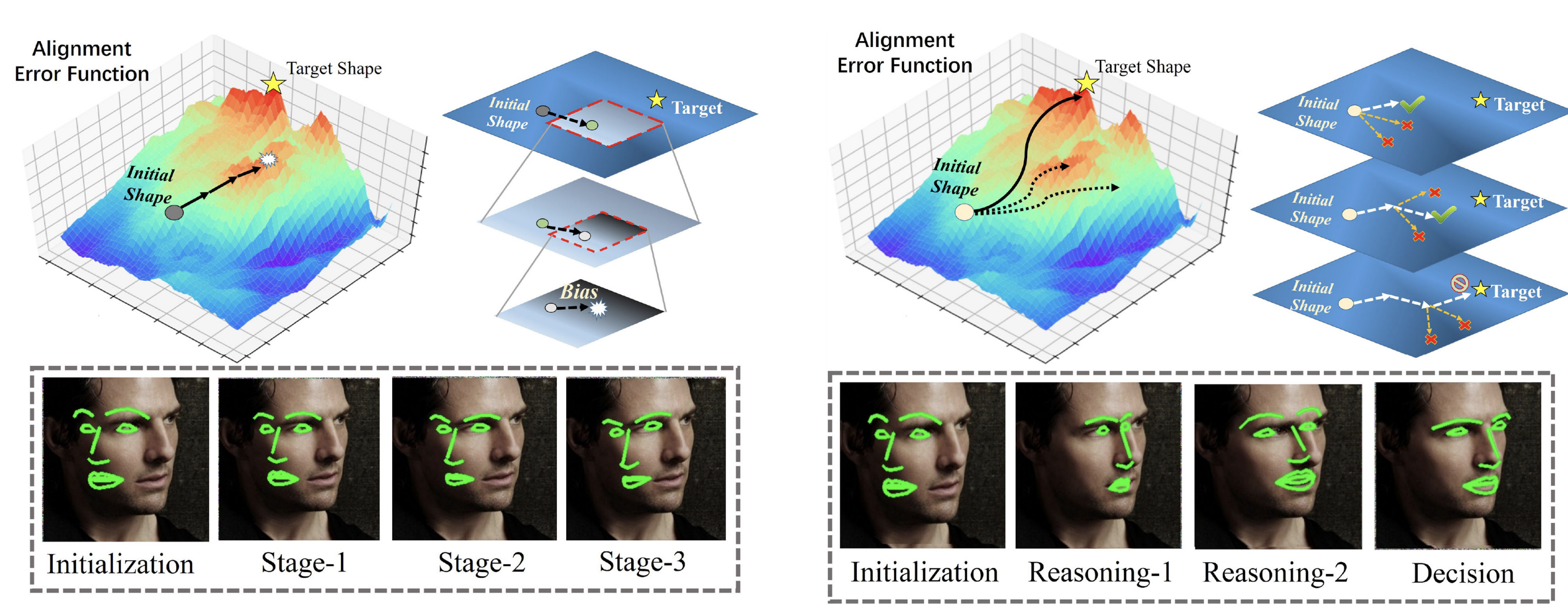
Education & Experiences
Ph.D. Student, Computational Design & Fabrication Group, CSAIL MIT, Sep. 2021 - Present
Advisor: Prof. Wojciech MatusikM.Phil. Student, The Chinese University of Hong Kong, Aug. 2019 - Aug. 2021
Advisors: Prof. Dahua Lin and Prof. Ziwei LiuVision Researcher, SenseTime Research, Aug. 2018 - July 2019
Advisors: Dr. Zhao Zhong, Dr. Wei Wu, and Dr. Junjie YanIntelligent Vision Group, Tsinghua University, Mar. 2017 - July 2018
Advisor: Prof. Jiwen LuB.Eng. in Automation, Tsinghua University, Aug. 2014 - July 2018